Introduction
When we think about modern medicine in the United States, it is easy to assume it is a beacon of progress that evolves daily. But what if you were told that some of its practices and metrics are rooted in outdated biases from centuries ago? The truth is that history has a way of influencing how we approach health and treatment even today. Hidden bias refers to unconscious attitudes or assumptions embedded in medical practices and systems that often stem from historical norms and continue to affect patient care. From gender disparities in research to biases in pain management, these subtle yet powerful forces shape how healthcare is delivered. Let’s explore seven surprising examples that reveal how American history's biases still impact modern medicine in the United States and consider what can be done to address them.
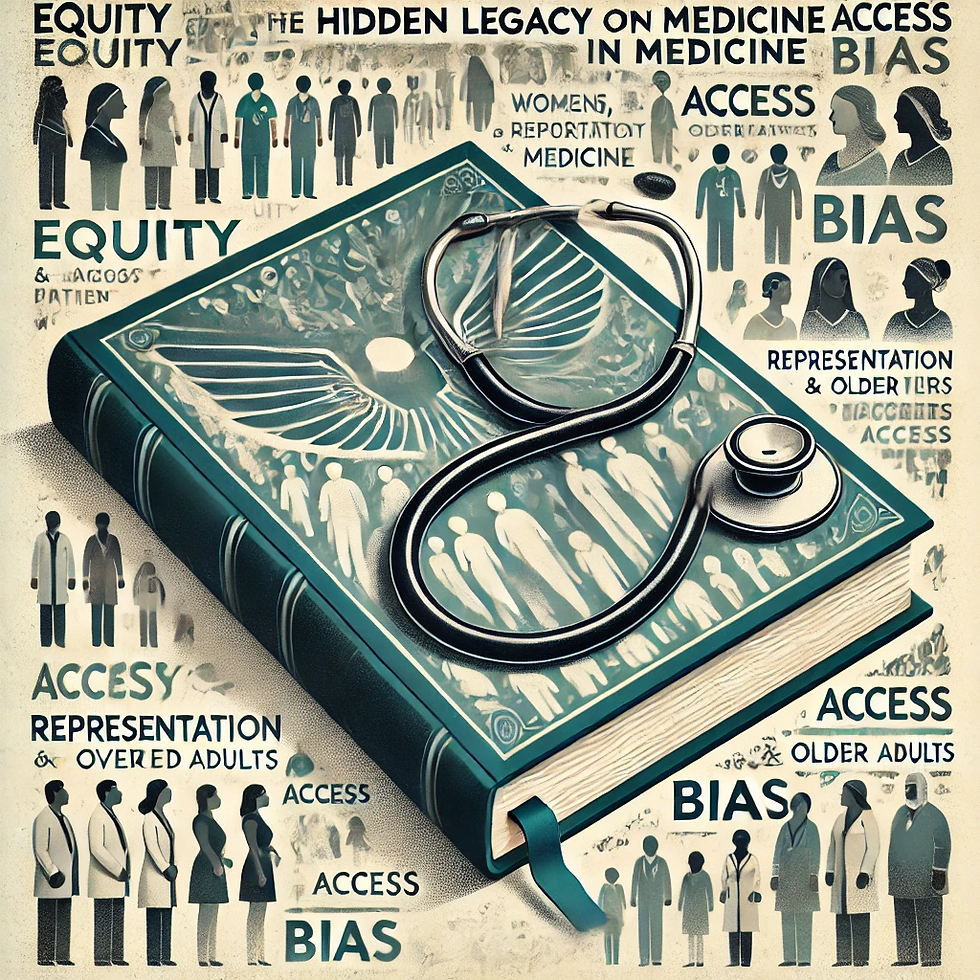
Medicine’s Top Hidden Biases
#7. BMI: An Old Metric with Modern Problems
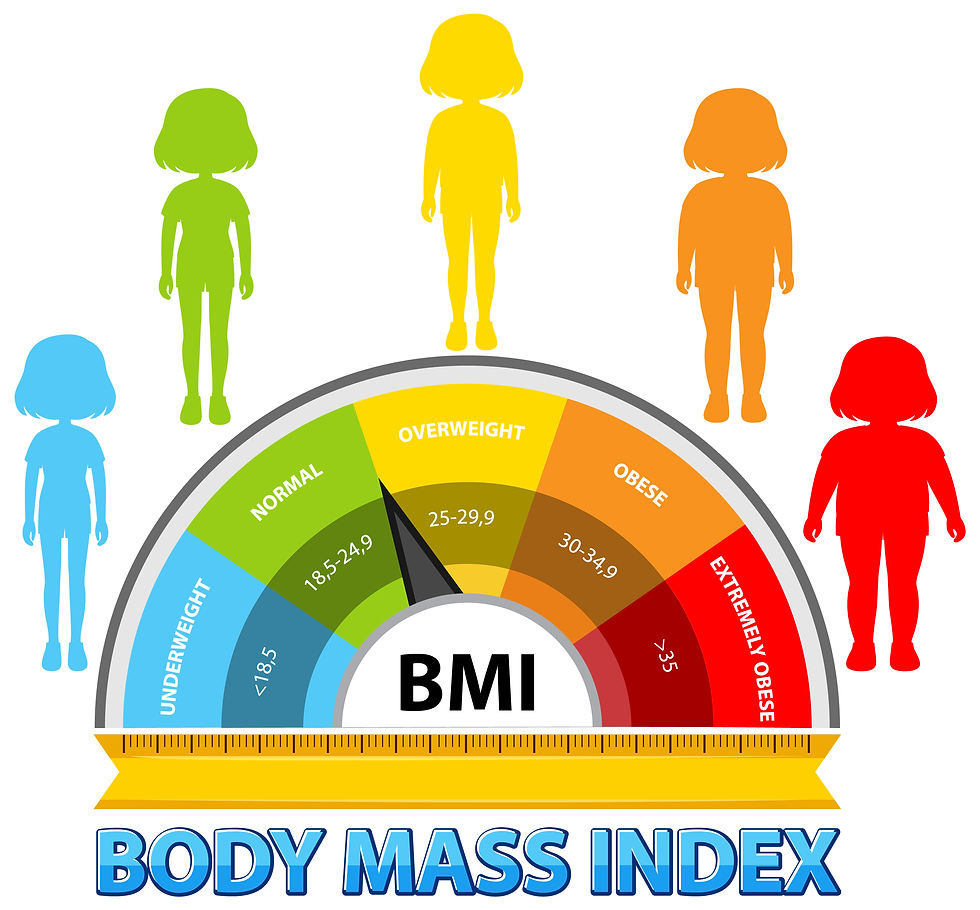
The History
BMI (Body Mass Index) was introduced in the 1830s by Adolphe Quetelet as a tool for studying population averages, not individual health. It was initially termed the “Quetelet Index” and was based on data from European men. This standard did not consider gender, racial, or cultural diversity. Later, the term "Body Mass Index" was introduced in 1972 by physiologist Ancel Keys. In his study that same year, Keys provided evidence to support its use and helped popularize the Quetelet Index in population-based research.
Modern Influence
BMI is still widely used in healthcare, insurance, and public health, even though it has limitations. BMI, as a sole criterion for health assessment, perpetuates inequities because it applies a one-size-fits-all approach to diverse populations.
Did You Know?
Professional athletes often fall into the "overweight" or even "obese" category due to their muscle mass, even though they are in peak physical condition. Conversely, people with "normal" BMI can still face significant health risks if they have high levels of visceral fat or poor metabolic health.
#6. Racial Bias in Pain Management
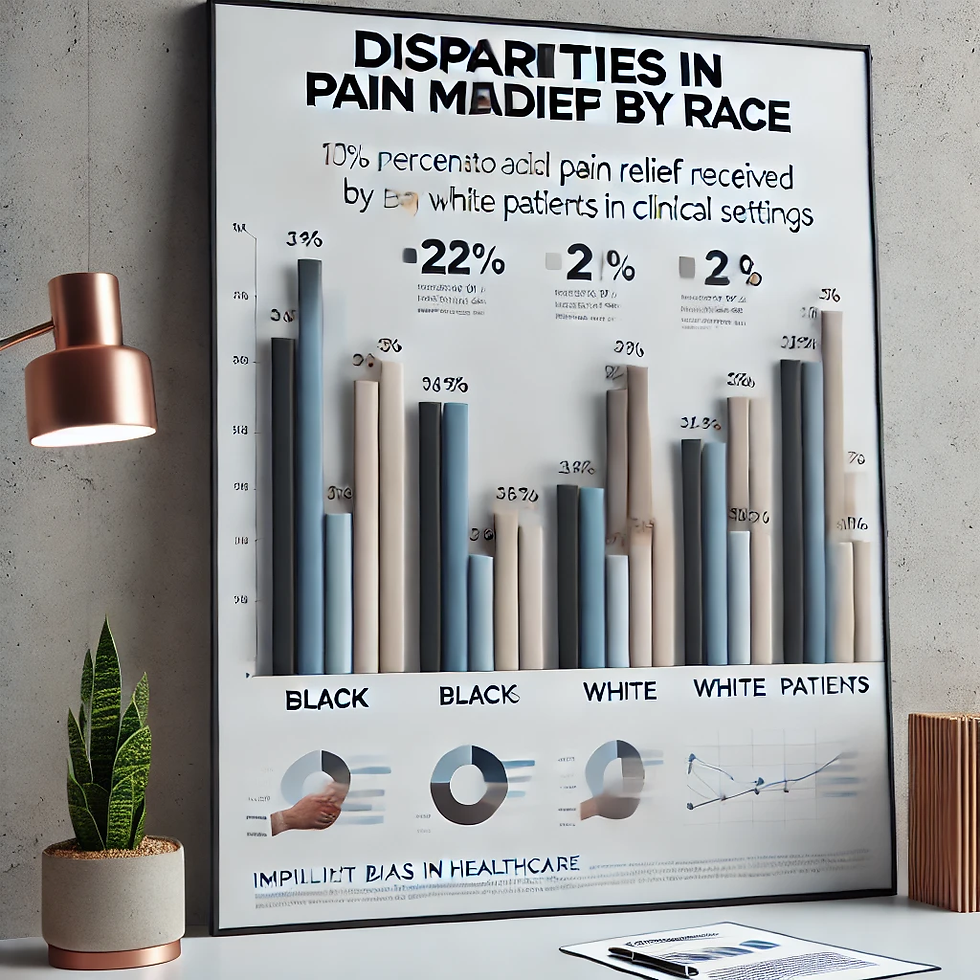
The History
An age-old myth claimed that Black individuals had higher pain thresholds. This false belief was rooted in slavery-era pseudoscience to justify the inhumane treatment of enslaved Black individuals. These ideas were embedded in early medical education and continued influencing healthcare practices.
Modern Influence
Research consistently shows that Black patients are less likely to receive adequate pain relief compared to white patients. Implicit bias among healthcare providers leads to assumptions that minimize Black patients’ reported pain levels while delaying or denying appropriate treatment. This bias persists in clinical settings and affects outcomes in post-surgical care, chronic pain management, and emergency medicine.
Did You Know?
A 2016 study revealed that some medical students and residents still believe myths about biological differences in pain perception between Black and white patients. This study underscores the need for better education to address implicit bias in medicine.
#5. Gender Bias in Diagnosing Conditions
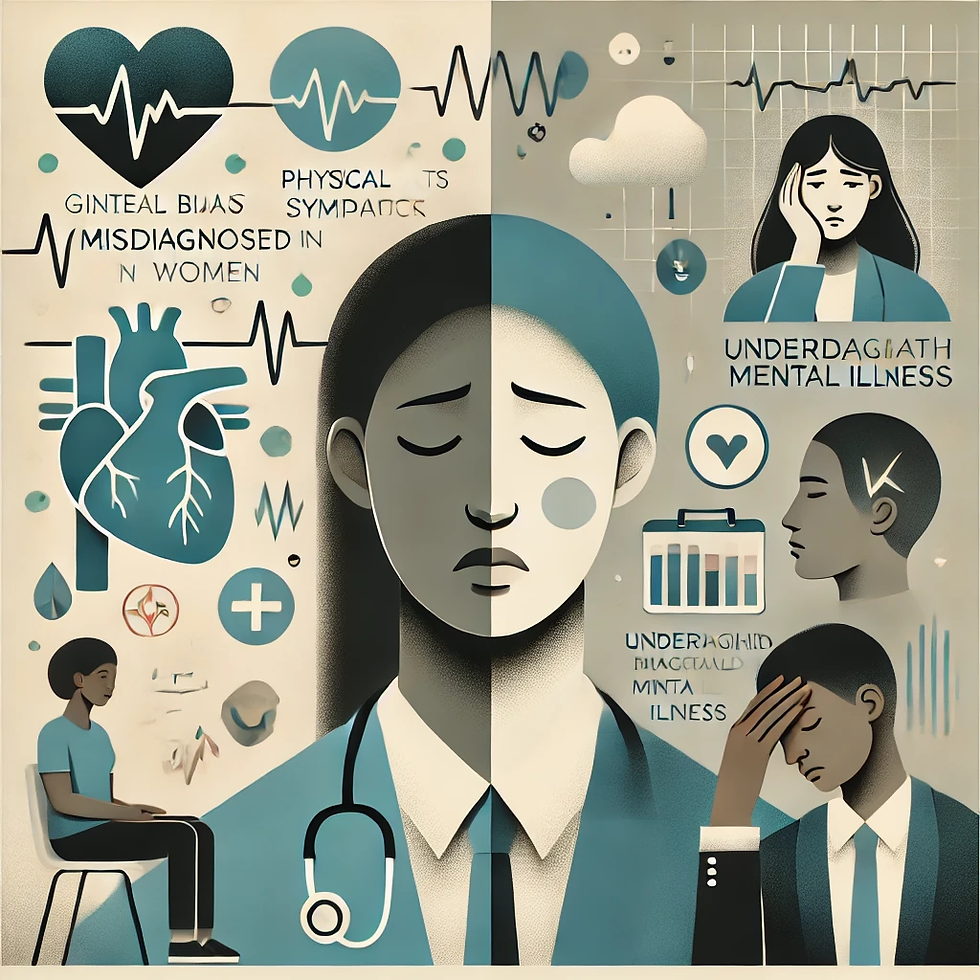
The History
Historically, medical research and education focused predominantly on men. As a result, women’s health was under-researched and misunderstood. Women were excluded from clinical trials until the 1990s because male physiology was a suitable proxy for all humans. Meanwhile, men’s emotional and mental health needs were often dismissed due to societal expectations of masculinity.
Modern Influence
Many conditions, such as heart disease, present differently in women but are still taught and diagnosed based on male-centric symptoms. For example, women experiencing heart attacks are more likely to report nausea, fatigue, or back pain rather than the classic chest pain typically associated with men. Similarly, men’s mental health needs are often overlooked due to societal pressures to appear strong or stoic. Depression in men, for example, may present as irritability or substance abuse rather than sadness, leading to missed or delayed diagnoses. Conditions like anxiety or eating disorders are also underdiagnosed in men because they are stereotypically viewed as “women’s issues.
Did You Know?
Heart disease kills more women annually than any other condition, yet it’s still considered a “man’s disease” in public perception. Conversely, men are four times more likely to die by suicide than women, partly due to underdiagnosis of mental health conditions.
#4. Socioeconomic Bias in Access to Care
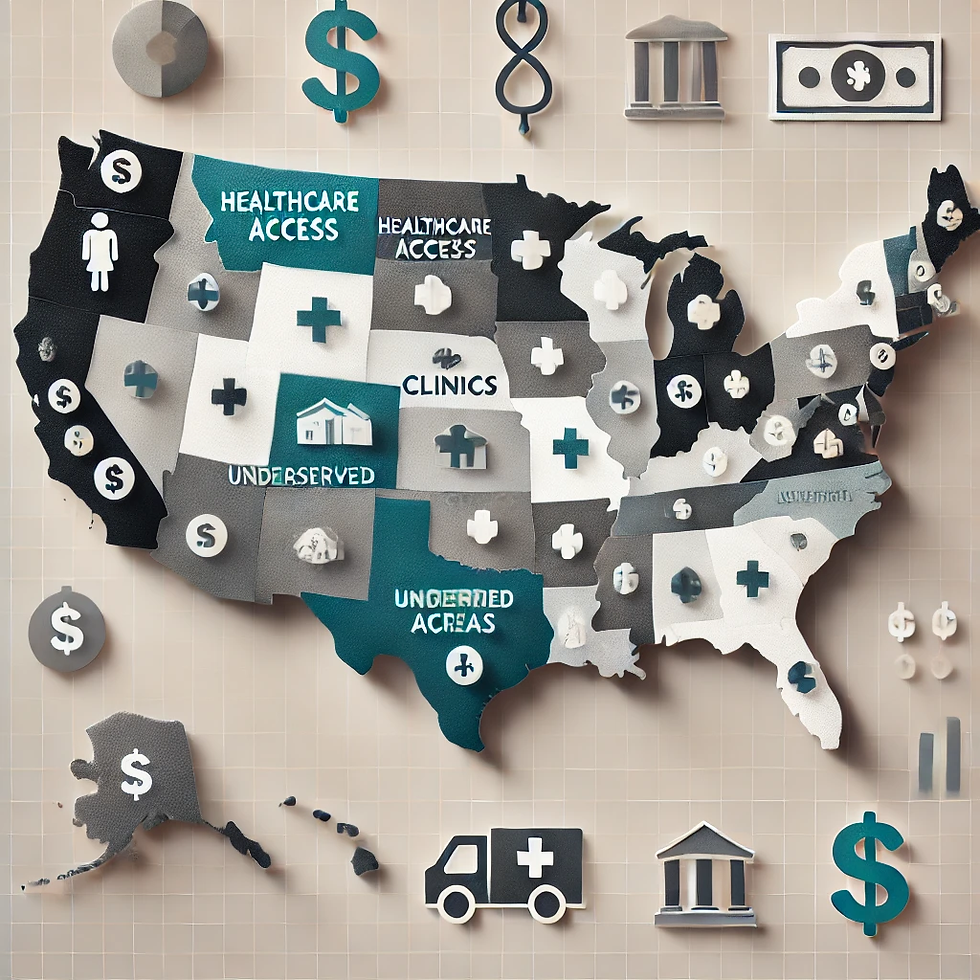
The History
In the early days, higher-quality healthcare systems were reserved for the wealthy who could afford private physicians or access to elite hospitals. Lower-income individuals were left reliant on overcrowded charity hospitals or, in many cases, had no access to medical care at all. This economic divide laid the foundation for systemic healthcare inequities that persist today.
Modern Influence
Wealth continues to play a significant role in determining access to quality care, including preventive screenings, advanced treatments, and specialized services. Underserved areas, often with higher proportions of low-income residents, face chronic shortages of healthcare resources, from clinics to qualified providers. Public health initiatives and safety-net programs have improved access somewhat, but disparities in care availability and quality remain.
Did You Know?
The term "postcode lottery" describes how healthcare quality and access can vary dramatically depending on where you live. Patients in wealthier zip codes can often access better services and shorter wait times than those in economically disadvantaged areas.
#3. Linguistic and Cultural Bias
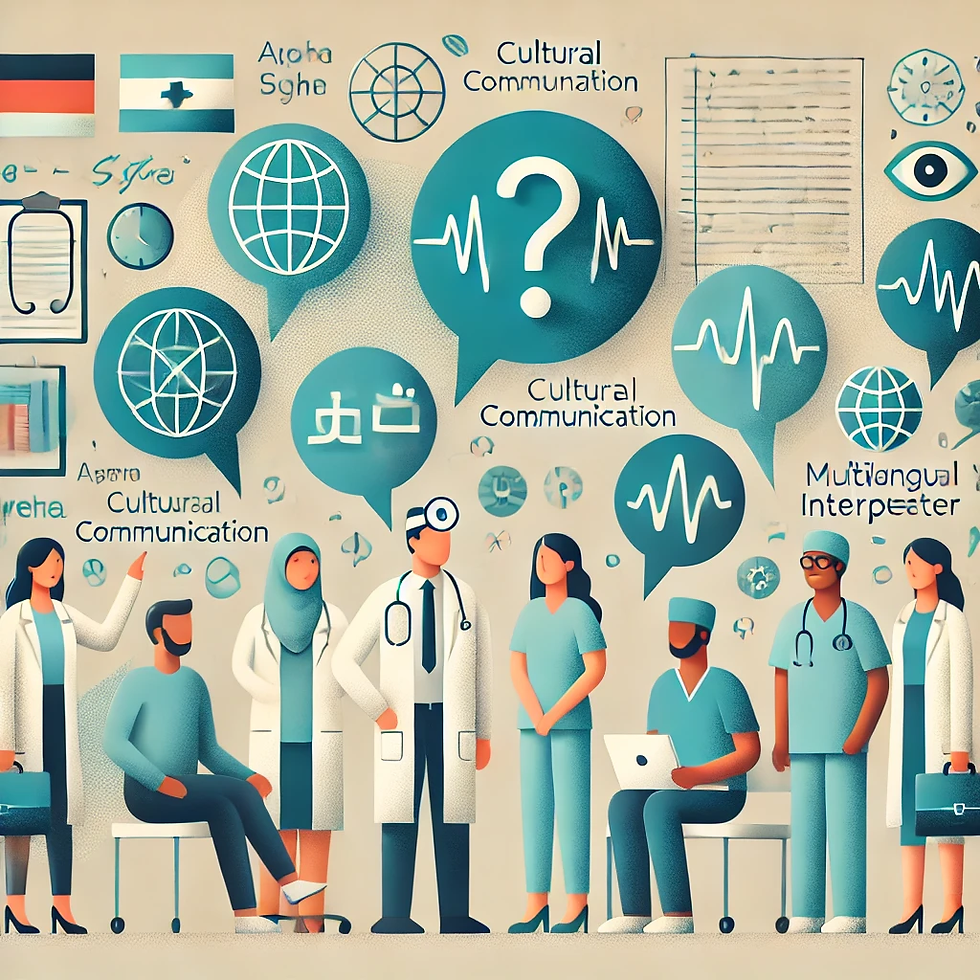
The History
During colonial eras, medicine in the United States dismissed indigenous and non-Western healing practices as primitive or unscientific and erased valuable cultural knowledge. Medical training and healthcare systems were built around the so-called “Anglo-American model” that excluded the traditions and needs of diverse populations. This disregard for cultural differences in healthcare sets the stage for systemic biases in how care is provided to non-Western communities.
Modern Influence
Language barriers continue to be a significant challenge in American healthcare. Patients with limited English proficiency often receive poor care that can significantly impact their health outcomes. Cultural insensitivity within the healthcare system can lead to miscommunication and misdiagnosis, and patients may also feel dismissed or misunderstood. Additionally, many providers may lack adequate training in cultural competence. As a result, treatment plans may fail to align with a patient’s cultural beliefs or practices, ultimately affecting the quality of care they receive.
Did You Know?
Studies show that offering care in a patient’s preferred language improves health outcomes. Yet many healthcare systems lack adequate access to interpreters or multilingual resources.
#2. Bias in Medical Research
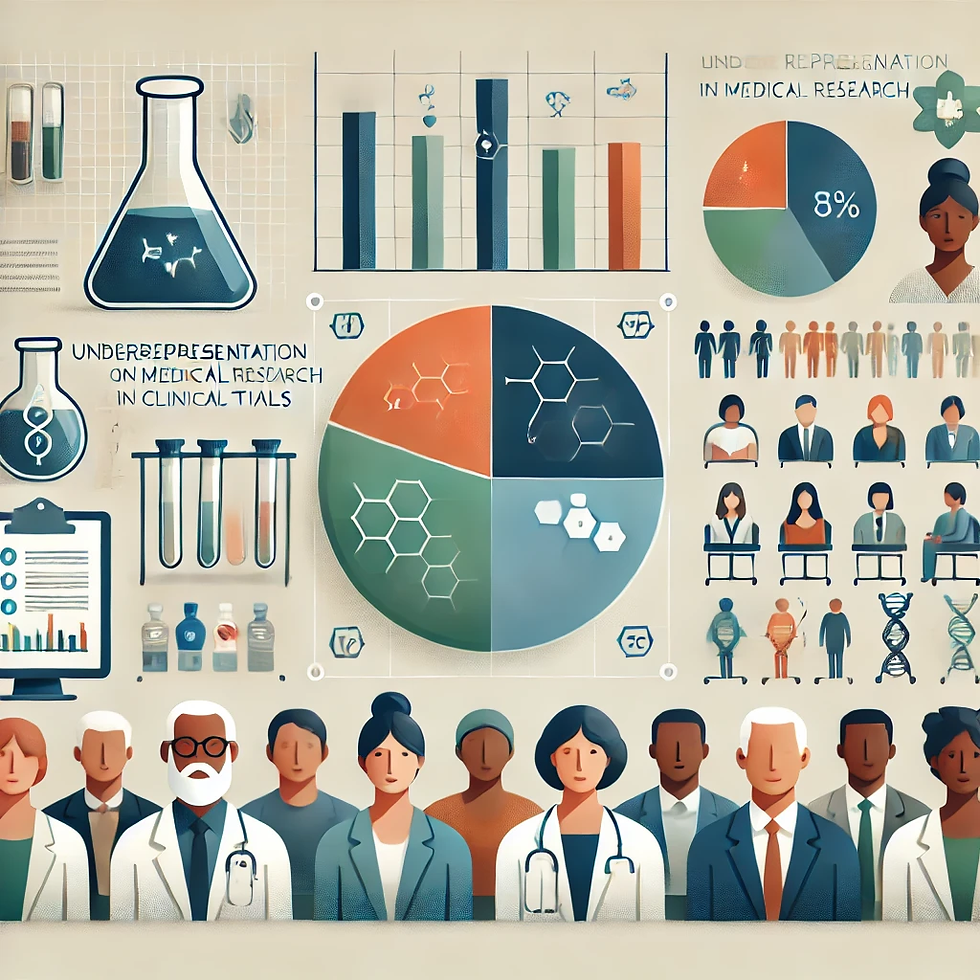
The History
For much of the 20th century, clinical trials primarily excluded women, racial minorities, and older adults. Women were often left out due to concerns about hormonal fluctuations and potential pregnancy risks. Racial minorities and older adults were underrepresented because of systemic biases and limited access to healthcare. As a result, treatments and guidelines were primarily developed for young white men. This approach failed to account for how these medical interventions might affect other groups and left significant gaps in healthcare outcomes.
Modern Influence
Many drugs and treatments remain less effective or harmful for populations not adequately represented in research. For example, some cardiovascular drugs are less effective in women because physiological differences were not considered during clinical trials. The lack of diversity in clinical research continues to create disparities in outcomes and limits the development of inclusive medical treatments. While efforts to diversify clinical trials are improving, progress has been slow. As a result, significant gaps remain in understanding how treatments impact different demographics.
Did You Know?
Older adultsaccount for most healthcare utilization globally and are consistently underrepresented in clinical trials. Despite making up more than 15% of the population in many countries, less than 10% of trial participants are over the age of 65, leading to potential gaps in understanding how drugs and treatments affect aging populations.
#1. Algorithmic Bias in AI Healthcare Tools
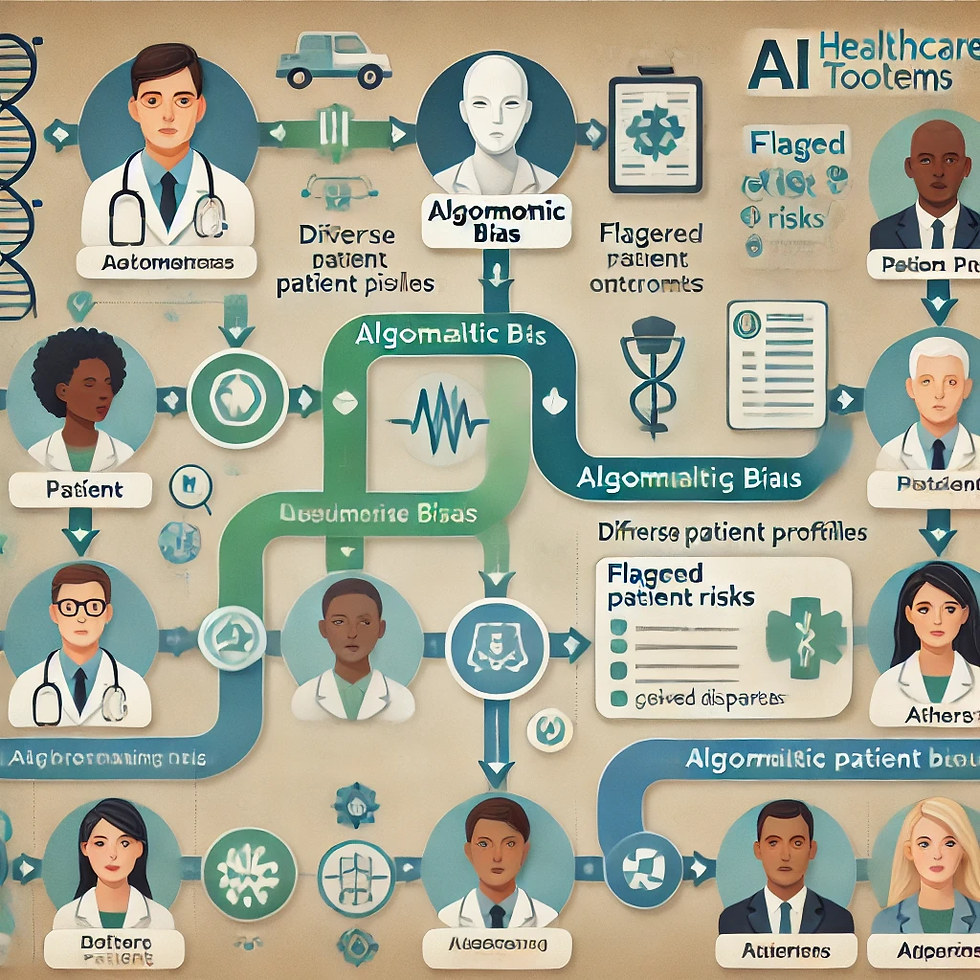
The History
Modern artificial intelligence (AI) models often rely on historical datasets that reflect systemic inequities from past healthcare and insurance practices. Early health and insurance data prioritized cost-saving measures instead of equitable care. As a result, modern AI systems inherit these biases and replicate them in decision-making processes. Many of these biases are rooted in outdated patterns, such as favoring younger, healthier individuals while penalizing those with complex medical histories. This creates challenges for ensuring that AI-driven healthcare systems provide fair and effective solutions for everyone.
Modern Influence
Biased AI algorithms are becoming more common in how insurers assess risk and determine coverage eligibility. These algorithms often deny care based on flawed or incomplete metrics. For example, patients with preexisting conditions or complex medical needs may be flagged as high-risk and have their access to comprehensive coverage limited.
These systems disproportionately affect individuals with chronic conditions, disabilities, or mental health needs, creating significant barriers to timely and effective care. Algorithms lack the nuance to understand and evaluate individual circumstances, and relying too heavily on AI without human oversight can increase inequities.
Did You Know?
In some cases, insurance companies use AI to flag patients for potential fraud or overutilization of services. This could result in unjust denial of coverage even for patients with legitimate medical needs.
Conclusion
The biases in modern American medicine may be historic, but their impact is still very much alive in modern medicine. From outdated metrics to systemic inequities, the remnants of the past continue to shape healthcare practices and outcomes today. Acknowledging these challenges is the first step toward creating a system that serves the population more fairly and effectively. By understanding these biases, we gain the power to address them and push for meaningful change. This means advocating for inclusive research, equitable policies, and culturally competent care. It’s about building a healthcare system that prioritizes fairness, representation, and the needs of all individuals, regardless of their background.
References
1977-1989 Timeline. (n.d.). Society for Women’s Health Research. https://swhr.org/about/1977-1989-timeline/
Abràmoff, M. D., Tarver, M. E., Loyo-Berrios, N., Trujillo, S., Char, D., Obermeyer, Z., Eydelman, M. B., & Maisel, W. H. (2023). Considerations for addressing bias in artificial intelligence for health equity. Npj Digital Medicine, 6(1), 1–7. https://doi.org/10.1038/s41746-023-00913-9
Adams, T. L. (2020). Health professional regulation in historical context: Canada, the USA and, the UK (19th century to present). Human Resources for Health, 18(1). https://doi.org/10.1186/s12960-020-00501-y
Affleck W, Carmichael V, Whitley R. Men's Mental Health: Social Determinants and Implications for Services. Can J Psychiatry. 2018 Sep;63(9):581-589. doi: 10.1177/0706743718762388. Epub 2018 Apr 19. PMID: 29673270; PMCID: PMC6109884.
Balch, B. (2024, March 26). Why we know so little about women’s health. Association of American Medical Colleges; Association of American Medical Colleges. https://www.aamc.org/news/why-we-know-so-little-about-women-s-health
Belenguer L. AI bias: exploring discriminatory algorithmic decision-making models and the application of possible machine-centric solutions adapted from the pharmaceutical industry. AI Ethics. 2022;2(4):771-787. doi: 10.1007/s43681-022-00138-8. Epub 2022 Feb 10. PMID: 35194591; PMCID: PMC8830968.
Bierer BE, Meloney LG, Ahmed HR, White SA. Advancing the inclusion of underrepresented women in clinical research. Cell Rep Med. 2022 Mar 7;3(4):100553. doi: 10.1016/j.xcrm.2022.100553. PMID: 35492242; PMCID: PMC9043984.
Business Standard. (2024, June 13). The role of BMI in health insurance: What you need to know. Business Standard. https://www.business-standard.com/content/specials/the-role-of-bmi-in-health-insurance-what-you-need-to-know-124061300863_1.html
Carland C, Hansra B, Parsons C, Lyubarova R, Khandelwal A. Adequate enrollment of women in cardiovascular drug trials and the need for sex-specific assessment and reporting. Am Heart J Plus. 2022 Jun 23;17:100155. doi: 10.1016/j.ahjo.2022.100155. PMID: 38559887; PMCID: PMC10978324.
Cedars-Sinai Medical Center. (2019, April 5). Time: Women Die From Heart Attacks More Often Than Men. Here’s Why — and What Doctors Are Doing About It. Time: Women Die from Heart Attacks More Often than Men. Here’s Why — and What Doctors Are Doing about It ; Cedars-Sinai Medical Center. https://www.cedars-sinai.org/newsroom/time-women-die-from-heart-attacks-more-often-than-men-heres-why--and-what-doctors-are-doing-about-it/
Celi LA, Cellini J, Charpignon ML, Dee EC, Dernoncourt F, Eber R, Mitchell WG, Moukheiber L, Schirmer J, Situ J, Paguio J, Park J, Wawira JG, Yao S; for MIT Critical Data. Sources of bias in artificial intelligence that perpetuate healthcare disparities-A global review. PLOS Digit Health. 2022 Mar 31;1(3):e0000022. doi: 10.1371/journal.pdig.0000022. PMID: 36812532; PMCID: PMC9931338.
Centers for Medicare and Medicaid Services. (2022). Building an Organizational Response to Health Disparities PROVIDING LANGUAGE SERVICES TO DIVERSE POPULATIONS: LESSONS FROM THE FIELD. https://www.cms.gov/About-CMS/Agency-Information/OMH/Downloads/Lessons-from-the-Field.pdf
Colón-Rodríguez, C. (2023, July 12). Shedding Light on Healthcare Algorithmic and Artificial Intelligence Bias | Office of Minority Health. Minorityhealth.hhs.gov. https://minorityhealth.hhs.gov/news/shedding-light-healthcare-algorithmic-and-artificial-intelligence-bias
Corliss, J. (2016, April 15). Understanding the heart attack gender gap - Harvard Health Blog. Harvard Health Blog. https://www.health.harvard.edu/blog/understanding-heart-attack-gender-gap-201604159495
Corliss, J. (2022, September 1). The heart disease gender gap. Harvard Health. https://www.health.harvard.edu/heart-health/the-heart-disease-gender-gap
Deering, M. (2023, September 29). Addressing language barriers in healthcare. Nursejournal.org. https://nursejournal.org/articles/language-barriers-in-healthcare/
Deering, S. (2022, April 18). 4 Myths about Black Patients and Chronic Pain. Www.healthcentral.com. https://www.healthcentral.com/pain-management/myths-black-patients-chronic-pain
Drwecki, B. B. (2015). Education to Identify and Combat Racial Bias in Pain Treatment. AMA Journal of Ethics, 17(3), 221–228. https://doi.org/10.1001/journalofethics.2015.17.3.medu1-1503
Espinoza, J., & Derrington, S. (2021). How Should Clinicians Respond to Language Barriers That Exacerbate Health Inequity? AMA Journal of Ethics, 23(2), 109–116. https://doi.org/10.1001/amajethics.2021.109
Flores, L. E., Frontera, W. R., Andrasik, M. P., del Rio, C., Mondríguez-González, A., Price, S. A., Krantz, E. M., Pergam, S. A., & Silver, J. K. (2021). Assessment of the Inclusion of Racial/Ethnic Minority, Female, and Older Individuals in Vaccine Clinical Trials. JAMA Network Open, 4(2), e2037640. https://doi.org/10.1001/jamanetworkopen.2020.37640
Gervasi, S. S., Chen, I. Y., Smith-McLallen, A., Sontag, D., Obermeyer, Z., Vennera, M., & Chawla, R. (2022). The Potential For Bias In Machine Learning And Opportunities For Health Insurers To Address It. Health Affairs, 41(2), 212–218. https://doi.org/10.1377/hlthaff.2021.01287
Gichoya JW, Thomas K, Celi LA, Safdar N, Banerjee I, Banja JD, Seyyed-Kalantari L, Trivedi H, Purkayastha S. AI pitfalls and what not to do: mitigating bias in AI. Br J Radiol. 2023 Oct;96(1150):20230023. doi: 10.1259/bjr.20230023. Epub 2023 Sep 12. PMID: 37698583; PMCID: PMC10546443.
Graley CE, May KF, McCoy DC. Postcode lotteries in public health--the NHS Health Checks Programme in North West London. BMC Public Health. 2011 Sep 28;11:738. doi: 10.1186/1471-2458-11-738. PMID: 21955810; PMCID: PMC3195760.
Gulati I, Kilian C, Buckley C, Mulia N, Probst C. Socioeconomic Disparities in Healthcare Access and Implications for All-Cause Mortality among US Adults: A 2000-2019 Record Linkage Study. Am J Epidemiol. 2024 Jul 24:kwae202. doi: 10.1093/aje/kwae202. Epub ahead of print. PMID: 39049439.
Gutin I. In BMI We Trust: Reframing the Body Mass Index as a Measure of Health. Soc Theory Health. 2018 Aug;16(3):256-271. doi: 10.1057/s41285-017-0055-0. Epub 2017 Oct 25. PMID: 31007613; PMCID: PMC6469873.
Heiat, A., Gross, C. P., & Krumholz, H. M. (2002). Representation of the Elderly, Women, and Minorities in Heart Failure Clinical Trials. Archives of Internal Medicine, 162(15). https://doi.org/10.1001/archinte.162.15.1682
Herrera AP, Snipes SA, King DW, Torres-Vigil I, Goldberg DS, Weinberg AD. Disparate inclusion of older adults in clinical trials: priorities and opportunities for policy and practice change. Am J Public Health. 2010 Apr 1;100 Suppl 1(Suppl 1):S105-12. doi: 10.2105/AJPH.2009.162982. Epub 2010 Feb 10. PMID: 20147682; PMCID: PMC2837461.
Hoffman KM, Trawalter S, Axt JR, Oliver MN. Racial bias in pain assessment and treatment recommendations, and false beliefs about biological differences between blacks and whites. Proc Natl Acad Sci U S A. 2016 Apr 19;113(16):4296-301. doi: 10.1073/pnas.1516047113. Epub 2016 Apr 4. PMID: 27044069; PMCID: PMC4843483.
Hoffman KM, Trawalter S, Axt JR, Oliver MN. Racial bias in pain assessment and treatment recommendations, and false beliefs about biological differences between blacks and whites. Proc Natl Acad Sci U S A. 2016 Apr 19;113(16):4296-301. doi: 10.1073/pnas.1516047113. Epub 2016 Apr 4. PMID: 27044069; PMCID: PMC4843483.
Improve patient outcomes with better language access. (2023, March 21). MedicalEconomics. https://www.medicaleconomics.com/view/improve-patient-outcomes-with-better-language-access
Institute of Medicine (US) Committee on the Future Health Care Workforce for Older Americans. Retooling for an Aging America: Building the Health Care Workforce. Washington (DC): National Academies Press (US); 2008. 2, Health Status and Health Care Service Utilization. Available from: https://www.ncbi.nlm.nih.gov/books/NBK215400
Jakubek, K. (2023, June 14). AMA adopts new policy clarifying role of BMI as a measure in medicine. American Medical Association. https://www.ama-assn.org/press-center/press-releases/ama-adopts-new-policy-clarifying-role-bmi-measure-medicine
Jin, X., Chandramouli, C., Allocco, B., Gong, E., Lam, C. S. P., & Yan, L. L. (2020). Women’s Participation in Cardiovascular Clinical Trials From 2010 to 2017. Circulation, 141(7), 540–548. https://doi.org/10.1161/circulationaha.119.043594
Jones, C. H., & Dolsten, M. (2024). Healthcare on the brink: navigating the challenges of an aging society in the United States. Npj Aging, 10(1), 1–10. https://doi.org/10.1038/s41514-024-00148-2
Kisacky, J. (2011). An Architectural History of US Community Hospitals. AMA Journal of Ethics, 21(3), 288–296. https://doi.org/10.1001/amajethics.2019.288
Koithan M, Farrell C. Indigenous Native American Healing Traditions. J Nurse Pract. 2010 Jun 1;6(6):477-478. doi: 10.1016/j.nurpra.2010.03.016. PMID: 20689671; PMCID: PMC2913884.
Lau, S. W. J., Huang, Y., Hsieh, J., Wang, S., Liu, Q., Slattum, P. W., Schwartz, J. B., Huang, S.-M., & Temple, R. (2022). Participation of Older Adults in Clinical Trials for New Drug Applications and Biologics License Applications From 2010 Through 2019. JAMA Network Open, 5(10), e2236149. https://doi.org/10.1001/jamanetworkopen.2022.36149
Lewis, J. H., Kilgore, M. L., Goldman, D. P., Trimble, E. L., Kaplan, R., Montello, M. J., Housman, M. G., & Escarce, J. J. (2003). Participation of Patients 65 Years of Age or Older in Cancer Clinical Trials. Journal of Clinical Oncology, 21(7), 1383–1389. https://doi.org/10.1200/jco.2003.08.010
Martin LA, Neighbors HW, Griffith DM. The experience of symptoms of depression in men vs women: analysis of the National Comorbidity Survey Replication. JAMA Psychiatry. 2013 Oct;70(10):1100-6. doi: 10.1001/jamapsychiatry.2013.1985. PMID: 23986338.
Mayo Clinic. (2022, January 20). How heart disease is different for women. Mayo Clinic. https://www.mayoclinic.org/diseases-conditions/heart-disease/in-depth/heart-disease/art-20046167
McGregor B, Belton A, Henry TL, Wrenn G, Holden KB. Improving Behavioral Health Equity through Cultural Competence Training of Health Care Providers. Ethn Dis. 2019 Jun 13;29(Suppl 2):359-364. doi: 10.18865/ed.29.S2.359. PMID: 31308606; PMCID: PMC6604769.
McKenzie SK, Oliffe JL, Black A, Collings S. Men's Experiences of Mental Illness Stigma Across the Lifespan: A Scoping Review. Am J Mens Health. 2022 Jan-Feb;16(1):15579883221074789. doi: 10.1177/15579883221074789. PMID: 35125015; PMCID: PMC8832600.
McMaughan DJ, Oloruntoba O, Smith ML. Socioeconomic Status and Access to Healthcare: Interrelated Drivers for Healthy Aging. Front Public Health. 2020 Jun 18;8:231. doi: 10.3389/fpubh.2020.00231. PMID: 32626678; PMCID: PMC7314918.
Mitchell, T. (2021, March 12). Algorithmic Bias in Health Care Exacerbates Social Inequities—How to Prevent It | Harvard T.H. Chan School of Public Health. Harvard T.H. Chan School of Public Health; HSPH. https://hsph.harvard.edu/exec-ed/news/algorithmic-bias-in-health-care-exacerbates-social-inequities-how-to-prevent-it/
Murphy, R. A., Ward, A., Larkin, J., McKeag, A., & O’Connor, M. (2024). Losing the Postcode Lottery: An Observational Study Examining the Association Between Patient Address and Delayed Discharge in a Rehabilitation Centre. Age and Ageing, 53(Supplement_4). https://doi.org/10.1093/ageing/afae178.016
National Institute on Minority Health and Health Disparities. (2024, January 12). Diversity and Inclusion in Clinical Trials. NIMHD. https://www.nimhd.nih.gov/resources/understanding-health-disparities/diversity-and-inclusion-in-clinical-trials.html
Native Medicine and Healing prior to the Reservation Era · Native Americans Then and Now. Nabb Research Center Online Exhibits. (n.d.). Libapps.salisbury.edu. https://libapps.salisbury.edu/nabb-online/exhibits/show/native-americans-then-and-now/reservations/medicine-and-healing-on-native
Nuttall FQ. Body Mass Index: Obesity, BMI, and Health: A Critical Review. Nutr Today. 2015 May;50(3):117-128. doi: 10.1097/NT.0000000000000092. Epub 2015 Apr 7. PMID: 27340299; PMCID: PMC4890841.
Pérez-Stable, E. J., & Webb Hooper, M. (2023). The Pillars of Health Disparities Science—Race, Ethnicity, and Socioeconomic Status. JAMA Health Forum, 4(12). https://doi.org/10.1001/jamahealthforum.2023.4463
Perspective | Is AI Making Claim Denials in Error? What Does It Matter if We Don’t Appeal? (2023, December 13). Apta.org. https://www.apta.org/article/2023/12/13/ai-and-appeals
Physicians for a National Health Program. (2010). Health Care Systems - Four Basic Models | Physicians for a National Health Program. Pnhp.org; Physicians For A National Health Program. https://www.pnhp.org/single_payer_resources/health_care_systems_four_basic_models.php
Pray R, Riskin S. The History and Faults of the Body Mass Index and Where to Look Next: A Literature Review. Cureus. 2023 Nov 3;15(11):e48230. doi: 10.7759/cureus.48230. PMID: 38050494; PMCID: PMC10693914.
Preez, V. du, Bennet, S., Byrne, M., Couloumy, A., Das, A., Dessain, J., Galbraith, R., King, P., Mutanga, V., Schiller, F., Zaaiman, S., Moehrke, P., & Heerden, L. van. (2024). From bias to black boxes: understanding and managing the risks of AI – an actuarial perspective. British Actuarial Journal, 29, e6. https://doi.org/10.1017/S1357321724000060
Reimagining Language Equity in Healthcare | Center for Bioethics. (2024, March 15). Umn.edu. https://bioethics.umn.edu/news/reimagining-language-equity-healthcare
Schoenthaler, A., & Williams, N. (2022). Looking Beneath the Surface: Racial Bias in the Treatment and Management of Pain. JAMA Network Open, 5(6), e2216281. https://doi.org/10.1001/jamanetworkopen.2022.16281
Schreiber, M. (2025, January 25). New AI tool counters health insurance denials decided by automated algorithms. The Guardian; The Guardian. https://www.theguardian.com/us-news/2025/jan/25/health-insurers-ai
Schwartz, J. B. (2023). Representative enrollment of older adults in clinical trials: the time is now. https://doi.org/10.1016/s2666-7568(23)00088-0
Scott PE, Unger EF, Jenkins MR, Southworth MR, McDowell TY, Geller RJ, Elahi M, Temple RJ, Woodcock J. Participation of Women in Clinical Trials Supporting FDA Approval of Cardiovascular Drugs. J Am Coll Cardiol. 2018 May 8;71(18):1960-1969. doi: 10.1016/j.jacc.2018.02.070. PMID: 29724348.
Scott, P. E., Unger, E. F., Jenkins, M. R., Southworth, M. R., McDowell, T.-Y., Geller, R. J., Elahi, M., Temple, R. J., & Woodcock, J. (2018). Participation of Women in Clinical Trials Supporting FDA Approval of Cardiovascular Drugs. Journal of the American College of Cardiology, 71(18), 1960–1969. https://doi.org/10.1016/j.jacc.2018.02.070
Shah HS, Bohlen J. Implicit Bias. [Updated 2023 Mar 4]. In: StatPearls [Internet]. Treasure Island (FL): StatPearls Publishing; 2025 Jan-. Available from: https://www.ncbi.nlm.nih.gov/books/NBK589697/
Sliwinski K, Ancheta AJ, Muir KJ, Lasater KB. Mapping Segregation Patterns of Hospital Care among Patients with Limited English Proficiency. J Immigr Minor Health. 2024 Dec;26(6):1113-1116. doi: 10.1007/s10903-024-01630-5. Epub 2024 Sep 13. PMID: 39269497; PMCID: PMC11606995.
Somashekhar, S. (2016, April 4). The disturbing reason some African American patients may be undertreated for pain. The Washington Post. https://www.washingtonpost.com/news/to-your-health/wp/2016/04/04/do-blacks-feel-less-pain-than-whites-their-doctors-may-think-so/
Starr, P. (2017). The Social Transformation of American Medicine. Basic Books.
Strother E, Lemberg R, Stanford SC, Turberville D. Eating disorders in men: underdiagnosed, undertreated, and misunderstood. Eat Disord. 2012;20(5):346-55. doi: 10.1080/10640266.2012.715512. PMID: 22985232; PMCID: PMC3479631.
Too much belly fat, even for people with a healthy BMI, raises heart risks. (2021, April 22). Www.heart.org. https://www.heart.org/en/news/2021/04/22/too-much-belly-fat-even-for-people-with-a-healthy-bmi-raises-heart-risks
Twersky SE, Jefferson R, Garcia-Ortiz L, Williams E, Pina C. The Impact of Limited English Proficiency on Healthcare Access and Outcomes in the U.S.: A Scoping Review. Healthcare (Basel). 2024 Jan 31;12(3):364. doi: 10.3390/healthcare12030364. PMID: 38338249; PMCID: PMC10855368.
Witt KA, Bush EA. College athletes with an elevated body mass index often have a high upper arm muscle area, but not elevated triceps and subscapular skinfolds. J Am Diet Assoc. 2005 Apr;105(4):599-602. doi: 10.1016/j.jada.2005.01.008. PMID: 15800563.