How Does Machine Learning Improve Brain Tumor Segmentation in MRI Scans?
- misshunzlazahid
- Jan 3
- 3 min read

"Segmentation of brain tumor MRI using machine learning" refers to the process of automatically identifying and outlining the boundaries of a brain tumor within a Magnetic Resonance Imaging (MRI) scan using machine learning algorithms, allowing for precise measurement and analysis of the tumor's size and location, crucial for diagnosis, treatment planning, and monitoring of brain tumors. Brain tumor segmentation is a medical image analysis task that involves the separation of brain tumors from normal brain tissue in magnetic resonance imaging scans. A collection of abnormal cells in the brain forming a mass is known as a Brain Tumor. A person’s brain is encased by a highly tough skull. Any type of increase in such a small location can produce complications. The death rate because of brain tumor is 76%, according to the International Agency of Research on Cancer.
MRI is a commonly used non-invasive technique of medical imaging that develops a variety and large number of contrasts of tissues in different modalities of imaging. It is used by medical specialists for the diagnosis of brain tumors on a large scale.
Magnetic resonance imaging (MRI) modalities are different ways of capturing characteristics of the body's anatomy in an MRI image. Each modality has a unique contrast and function. The most used MRI modalities are:
T1 image sequence (T1)
Contrast enhanced T1 weighted sequence with contrast agents of gadolinium (T1Gd)
T2 image sequence
FLAIR—fluid-attenuated inversion recovery sequence
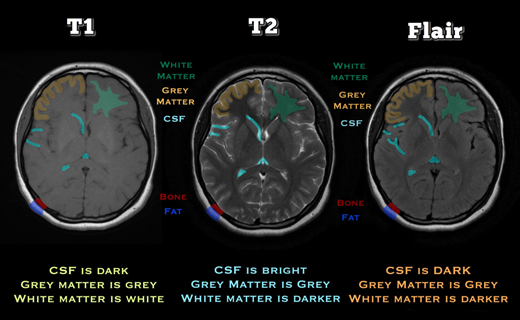
The distinct sub-regions of tumor predicted using MR imaging are as follows:
Enhancing tumor
Non-enhancing tumor
Necrotic tumor
Peritumoral edema
The segmentation of the tumor manually, then its analysis using its structural MRI images, is very difficult and time-consuming and requires a professional neuroradiologist. In field of medical imaging, automating the segmentation of brain tumor is also very complicated. Segmentation of brain tumor through MRI scan is considered a necessary process for the diagnosis of brain tumor and it's treatment. To make therapy progress more successful and relevant, radiologists can use an automated technique of segmentation of lesions that helps them give critical information regarding the location, shape, and volume of the tumors. Size, bias field (undesirable artefact owing to faulty picture capture), location, and form are only a few of the variations in the tumor and the normal tissues near it that make segmentation in medical imaging analysis difficult. Several approaches have been implemented in the literature to determine the accurate and precise boundaries of brain tumor in the images.
Manually extracted features are most used in machine learning algorithms to address the above-mentioned issues (or pre-defined features). First, the crucial aspects are extracted from the input images by using a method of feature extraction in segmentations like this, and after that, a specific model is trained that can differentiate the tumor and the normal tissues. Manually extracted features are used along with various other brain tumor classifiers, such as random forest, fuzzy clustering, and support vector machine in most commonly designed algorithms of machine learning. It is very time-consuming to extract features and details related to edges and the remaining relevant information using feature extraction algorithms and designed methodologies to extract features, edge-related details, and other relevant information. Additionally, such approaches fail in the cases in which the borders between the malignancies and the healthy tissues are blurry and ambiguous. Many approaches were developed for the MR images that are annotated automatically by training a CNN architecture for categorizing with respect to sequences in 2D perspective in order to avoid high memory and computational costs in 3D convolutions. Both of these works, however, share some flaws that make them incomparable.
References:
1. Black, P.M., Brain tumors. New England Journal of Medicine, 1991. 324(22): p. 1555-1564.
2. Zülch, K.J., Brain tumors: their biology and pathology. 2013: Springer-Verlag.
3. Herholz, K. Brain tumors: an update on clinical PET research in gliomas. in Seminars in nuclear medicine. 2017. Elsevier.
4. Butowski, N.A., Epidemiology and diagnosis of brain tumors. CONTINUUM: Lifelong Learning in Neurology, 2015. 21(2): p. 301-313.
5. Jain, R., Perfusion CT imaging of brain tumors: an overview. American Journal of Neuroradiology, 2011. 32(9): p. 1570-1577.
6. Young, G.S., Advanced MRI of adult brain tumors. Neurologic clinics, 2007. 25(4): p. 947-973.
7. Mohan, G. and M.M. Subashini, MRI based medical image analysis: Survey on brain tumor grade classification. Biomedical Signal Processing and Control, 2018. 39: p. 139-161. Assessed and Endorsed by the MedReport Medical Review Board